When the hackers get hacked
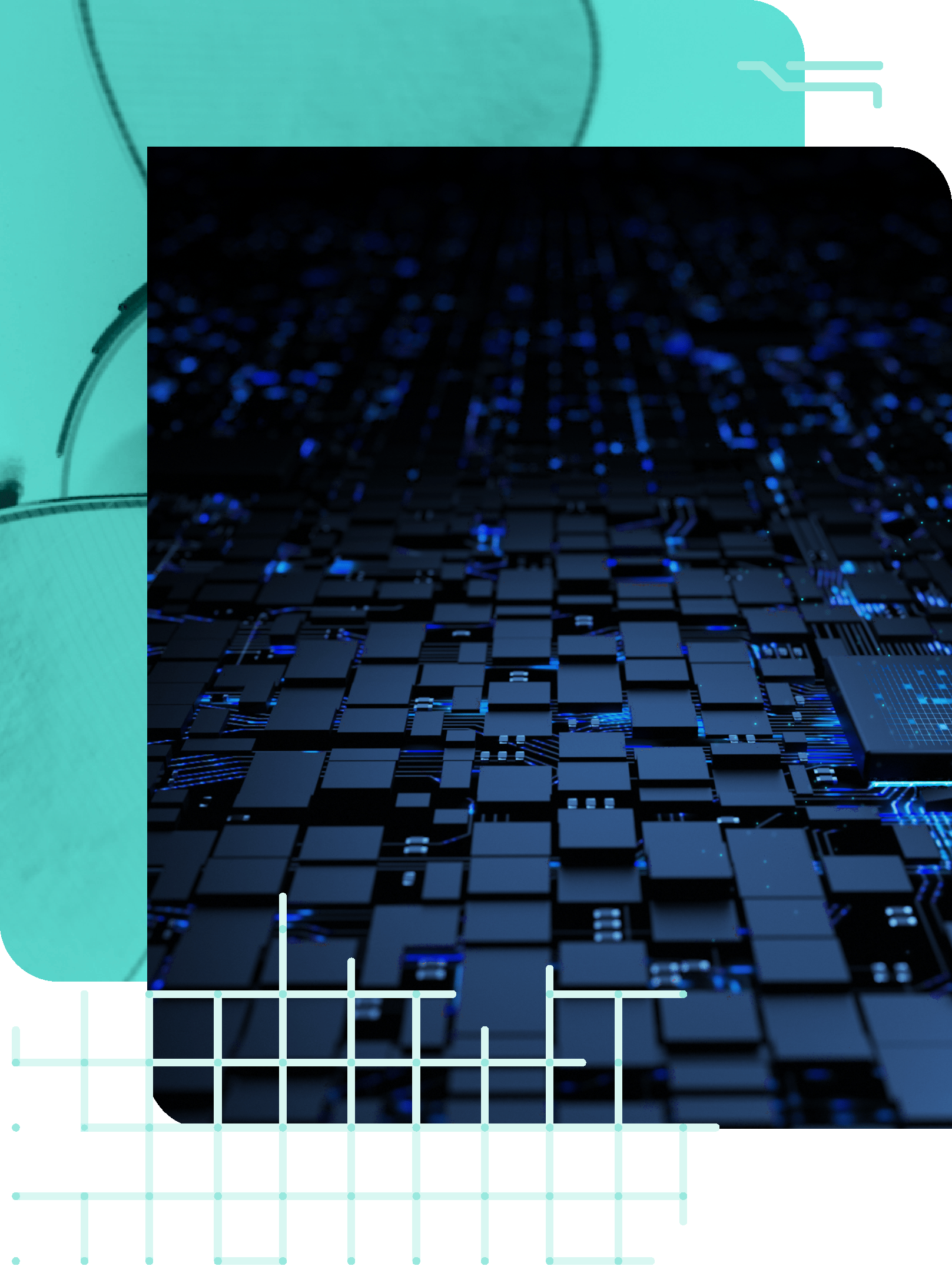
Blog
When the hackers get hacked: part 2
Blog written by Noël Keijzer, Cyber security expert at the Northwave CERT
In the previous blog, we presented the contents and origins of the leaked data related to Conti ransomware gang [1]. In addition to that, we translated the entire dataset and made it publicly available to the security community [2]. In this blog, we dive into details of the internal conversation among Conti gang actors. Our goal in this blog is to show what meaningful information we can subtract from the data. For this reason, we do not display all possible findings. Instead, we focus on the significant (most frequent) information. We acquired this information from an analysis of leaked files [3]: “Conti Chat Logs 2020.7z” and “Conti Jabber Chat Logs 2021 - 2022.7z”. These two files compress hundreds of thousands of internal conversations from 2020 till 2022. The analysis in this blog is intended to provide answers to the following questions:- What is in the data (an overview of the communication)?
- What roles are played by actors in the Conti gang?
- Can we identify the real identity of the Conti actors?
- What IP addresses and URLs (HTTP and HTTPS) are part of Conti’s infrastructure?
- How many Conti victims are there and who are they?
1. What is in the data (an overview of the communication)?
After uncompressing the two leaked files (“Conti Chat Logs 2020.7z” and “Conti Jabber Chat Logs 2021 - 2022.7z”) it showed 544 .json files. After those files were merged, we’ve observed almost 169k records dating from 21/06/2020 till 02/03/2022. Overall, the data containing internal chats between actors of the Conti gang. It is important to stress that it doesn’t contain direct conversations between Conti actors and their victims. However, we’ve found some conversations in which Conti actors pasted snapshots of their conversation with a victim. On the following table we summarize the overall information of the data.# | File name | Content | Records | First record | Last record | Unique users |
1 | Conti Chat Logs 2020.7z | 148 files | 107.967 | 2020-06-21 | 2020-11-16 | 465 |
2 | Conti Jabber Chat Logs 2021 - 2022.7z | 396 files | 60.773 | 2021-01-29 | 2022-03-02 |
After plotting the number of messages exchanged per day, depicted in the graph below, we noticed three gaps: (1) from 17/11/2020 to 18/01/2021, (2) on 07/02/2021 and (3) on 13/02/2021. The reason for the first gap is mainly related to law enforcement actions [4], according to Brian Krebs, a security journalist. The other two gaps still have an unclear explanation. On average, actors in the chats sent 272 messages per day. Conversations in 2020 were clearly more frequent than in 2021 and 2022. Interestingly, on 23/07/2020, there was a peak of 2465 messages. Northwave is still investigating the reason for peaks in the entire dataset.
On average, actors in the chats sent 272 messages per day. Conversations in 2020 were clearly more frequent than in 2021 and 2022. Interestingly, on 23/07/2020, there was a peak of 2465 messages. Northwave is still investigating the reason for peaks in the entire dataset.
2. What roles are played by actors in the Conti gang?
Our analysis discovered 465 distinct actors. This section classifies the top ten actors who sent and received the most messages. In addition to the top ten, we decided to include the actor who occupies position 13 (bio). The reason for this is that, while 150 actors were active for more than one year, bio was active for only 49 days. Despite this, bio was already among the top actors. Following, we describe how we manually inferred the role of each of the leading actors. Please note, whenever we write “he”, “she” can also be read.
# | Actors | Total messages | First Message | Last Message | Diff Days | Inferred Role |
1 | target | 36648 | 21/06/2020 | 22/10/2021 | 487 | Human resource manager |
2 | bentley | 36465 | 22/06/2020 | 01/03/2022 | 617 | Manager (and tester) of ransomware |
3 | stern | 28581 | 21/06/2020 | 21/02/2022 | 609 | Task manager |
4 | defender | 20379 | 22/06/2020 | 28/02/2022 | 616 | Systems administrator |
5 | hof | 11164 | 22/06/2020 | 29/12/2021 | 555 | Programmers’ manager |
6 | deploy | 8955 | 22/06/2020 | 22/10/2021 | 487 | Programmer of ransomware (crypto) |
7 | driver | 7892 | 28/10/2020 | 22/02/2022 | 482 | Operation lead programmers |
8 | mango | 7557 | 21/06/2020 | 02/03/2022 | 618 | General manager |
9 | mushroom | 7162 | 22/06/2020 | 01/11/2021 | 496 | Programmer |
10 | professor | 6565 | 22/06/2020 | 17/01/2022 | 574 | Senior developer and tester |
… | … | … | … | … | … | … |
13 | bio | 5367 | 02/11/2021 | 21/12/2021 | 49 | Negotiator |
1.Target. This actor proved to be one of the most difficult to determine the role of in the gang, because of the message volume. From his writing and statements, we arrive at several conclusions. First, we concluded he manages the testing department based on a message where he said, “[…] I am building a testing department […].” However, we are most certain on inferring that target is a manager of the human resources. We unravelled that since multiple actors talked to him regarding hiring. For example, salamandra said to target: “I am looking for candidates via mail on HH”; viper says to target “salary depends on who I am looking for standard schedule from 9 - 6 online payment 2 times a month on the card”. Target sent messages to 38 distinct users and received messages from 39 other distinct users.
2. Bentley. After analysing messages from/to bentley, we concluded he is a Ransomware Binaries and Tester manager. We deduced that because he wrote chats to the actor deploy like: “Please make 1 crypt gi11.dll and da11.dll for Tilar”, “Please make a loader dll crypt with a simple icon”, “Please make more crypts for Merch”, and “Please make a crypt gi6.dll for Tilar”. Bentley also asks to deploy the creation of lockers on behalf of other actors (the actors tilar and merch). It is clear that bentley also tests the ransomware programs, this finding is based on the following chat: “Yes. I am a tester. I pass it on to Deploy and Marcel and then test the result. And give to clients. Or whoever orders”, “[…] I am a tester. I test and issue them to clients”, and “Will be your tester from tomorrow”. Bentley sent messages to 105 distinct users and received messages from 97 other distinct users.
3. Stern. After analysing messages from and to Stern, we concluded he is one of the big bosses and a task manager. We extrapolated that because he wrote, “handed out tasks to everyone here, as I see everything”. He receives messages of actors asking for instructions, for example, the message from ceram: “Good afternoon. I am a new employee. Please instruct me.” Stern also wrote: “I am a cashier”. Thus, he has access to the money of the group. Also, Stern sent messages to 233 distinct users and received messages from 152 other distinct users. This makes Stern one of the people that interacted with most actors in the data.
4. Defender. After analysing messages from and to defender, we concluded that he is the System Administrator. We inferred that because he reminded the bulk of the users to share their Jabber credentials. Also, defender receives questions on accounts and access to specific panels, such as (from stern) “give access to the mavemat project which is the proton checker bend” and (from viper): make a test acc for new admins”, (from salamandra): “need acc for new programmer”. Defender sent messages to 326 distinct users and received messages from 148 distinct users.
5. Hof. After analysing messages from and to hof, we concluded he is the manager of the programmers. We figured that because he received messages such as (from xoc) “Hello. I am a new programmer. I was told to ask you for assignments.” Hof sent messages to 51 distinct users and received 51 messages from distinct users.
6. Deploy. After analysing messages from/to deploy, we concluded that he is a programmer focused on cryptography (the ransomware). We deduced that since he receives requests from bentley to create binaries for other actors with antivirus (AV) obfuscation through cryptography. Deploy wrote to target: “in general, yes. Crypt largely solves the problem of signature detection”. From this, we deduce that in this case, ‘crypt’ refers to AV-evasion. Deploy sent messages to 30 distinct users and received messages from 26 distinct users.
7. Driver. After analysing messages from and to driver, we concluded that he resembles the operational lead of programmers. We inferred that, because he is involved with the programmers on a lower level. He reminds people of their tasks, for example when he wrote: “you made a commitment on Thursday that you would finish by tonight. Then he unilaterally violated it and wrote what you would do on Fri.[..] Where is the outcome? How are we going to work with you?” and “Ok, there you have another small task to change the width of the field”. Also, he corrects the code of programmers, as he wrote: “+= means a = a + b; // You didn’t specify the field in the selection that you have in the output”, “Hello, I corrected the task code a little there, uploaded it to git, look too. Such a question, does it work for you?” and “it is not necessary to look for the cause by modules. I found it. The hammer will appear to test.” Driver sent messages to 24 distinct users and received messages from 20 distinct users.
8. Mango. After analysing messages from and to mango, we concluded that he is a general manager. We deduced that as he mentioned: “I am a general manager, if you need to contact me”. Mango sent messages to 129 distinct users and received 125 messages from distinct users.
9. Mushroom. After analysing messages from and to Mushroom, we concluded that he is a programmer. We discovered that, because he wrote memos on code changes, such as "I updated the bot in the admin panel, yesterday there was version 107, with non-working addresses. ". Also, in a conversation with Target where Target asked Mushroom about programmer's recruitment advice, Mushroom wrote, "I didn't come by myself, they found me through an ad. See what this RingCentral consists of (what languages and libraries are used in it), based on this, look for a person who worked with these libraries and knows these languages, come up with a test task based on this [ ...] It is better that these people have at least some open source projects on github or other places. Even better, they should be able to program on different platforms (Windows and Linux) at least." Mushroom sent messages to 20 distinct users and received messages from 20 distinct users
10. Professor. After analysing messages from and to professor we concluded he is a Senior Developer and Tester. We unveiled this since he received messages requesting him to test things, such as from Dorius: “Hello. on Monday you will need to test the shellcode to check the otstuk, there will be a check on the incodes that take the executable”. Also, he received the message, seemingly sent to all programmers: “Hi all. To all developers, including the web, please send me a short list of your skills by programming languages and stacks. Thanks.” Professor is a Senior which became clear from the following message from Target: “you are their teacher mentors” and “you are wikipedia for them”. Professor sent messages to 48 distinct users and received messages from 51 distinct users
13. Bio. After analysing messages from and to bio, we concluded he is a negotiator who also analyses the value of data extracted from victims. We discovered that based on dozens of statements, for example: “I think we will press up to 1.5k”, “I made a 25% discount and they will pay within 48 hours”, and “I have a mountain of information to analyse”. Bio sent messages to 8 distinct users and received messages from 8 distinct users. 80% of his messages were exchanged with skippy (his negotiator friend) and tramp (his manager). The take-away message of this section is that by reading chats, we can identify the roles of most actors. Besides the top actors, we identified a list of actors under the management of other actors. For example, by recognising the programmers’ manager, we could compose a list of programmers. The same applies to the manager of the negotiators, the manager of the ransomware developers and others. Based on these inferences, we were able to identify more than half of the actors in the leaked chat. It was harder to classify actors with minimal interaction. Overall, we were impressed by the organisation and the size of the Conti gang. These aspects justify our observations on the number of victims the group could manage simultaneously.
3. Can we identify the real identity of the actors in the data?
We identified 1076 different email addresses in the communication. We’ve filtered out emails from protonmail.com and with .onion aiming at finding personal emails (for attribution purpose). After this filter 652 emails remain and will be discussed in this section.
# | Email address | Freq. |
1 | DorothyStewartkaPq@yahoo.com | 66 |
2 | HardenKidd49@yahoo.com | 62 |
3 | HennemanFern4@yahoo.com | 62 |
4 | goldcoin@exploit.im | 57 |
5 | nodex08@tutanota.com | 54 |
6 | dictyna@tssssss.info | 53 |
7 | Vakomsyurebf@yahoo.com | 52 |
8 | Kasazhtiklon@yahoo.com | 52 |
9 | Chaadlinonzh@yahoo.com | 52 |
10 | Schatodalsaz@yahoo.com | 52 |
After filtering, the top 10 addresses have a very similar syntax (especially the yahoo addresses). Three of these emails HennemanFern4@yahoo.com, Kasazhtiklon@yahoo.com, and Schatodalsaz@yahoo.com are listed as part of TrickBot in a “notice of hearing re plaintiffs’ motion” [5]. We observed that these emails have a close relation. Each of these emails are part of conversations between ganesh and stern, in which the former updates the latter on the list of credentials used to obtain access to their infrastructure. These messages are a copy/paste of a table (because it contains \n and \t in a structured way). A snapshot of one message is the following:
root | 62.108.35.164 | 34fhjdgEN3rE5ff | DE | https://germanvps.com | Kasazhtiklon@yahoo.com | GKcM6PMLI5wi | 4 |
root | 185.234.72.94 | 34𝑓ℎ𝑗𝑑𝑔𝐸𝑁3𝑟𝐸5𝑓𝑓 | DE | https://𝑑𝑒𝑖𝑛𝑠𝑒𝑟𝑣𝑒𝑟ℎ𝑜𝑠𝑡.𝑑𝑒 | Kasazhtiklon@𝑦𝑎ℎ𝑜𝑜.𝑐𝑜𝑚 | GKcM6𝑃𝑀𝐿𝐼5𝑤𝑖 | 4 |
root | 51.89.163.33 | 34fhjdgEN3rE5ff | UK | https://www.vpsroom.com | Kasazhtiklon@yahoo.com | GKcM6PMLI5wi | 5 |
root | 107.155.137.28 | 34𝑓ℎ𝑗𝑑𝑔𝐸𝑁3𝑟𝐸5𝑓𝑓 | US | www.𝑡𝑟𝑢𝑛𝑘𝑠𝑝𝑎𝑐𝑒ℎ𝑜𝑠𝑡𝑖𝑛𝑔.𝑐𝑜𝑚 | Kasazhtiklon@𝑦𝑎ℎ𝑜𝑜.𝑐𝑜𝑚 | GKcM6𝑃𝑀𝐿𝐼5𝑤𝑖 | 5 |
root | 194.5.249.248 | z0OjS6l2B8MPgIke37 | RO | https://cryptoho.st/ | Kasazhtiklon@yahoo.com | GKcM6PMLI5wi | 7 |
root | 195.123.241.136 | P1𝑁𝑅𝑖81𝑈5𝑣𝑠𝑆 | NL | https://ℎ𝑜𝑠𝑡−𝑓𝑜𝑟.𝑛𝑒𝑡 | Kasazhtiklon@𝑦𝑎ℎ𝑜𝑜.𝑐𝑜𝑚 | GKcM6𝑃𝑀𝐿𝐼5𝑤𝑖 | 5.10 |
root | 185.99.2.179 | TWykiXbxel | BA | https://www.centohost.com | Kasazhtiklon@yahoo.com | GKcM6PMLI5wi | 5 |
root | 5.182.211.25 | 34𝑓ℎ𝑗𝑑𝑔𝐸𝑁3𝑟𝐸5𝑓𝑓 | DE | https://𝑚𝑦.𝑓𝑙𝑎𝑢𝑛𝑡7.𝑐𝑜𝑚 | Kasazhtiklon@𝑦𝑎ℎ𝑜𝑜.𝑐𝑜𝑚 | GKcM6𝑃𝑀𝐿𝐼5𝑤𝑖 | 5 |
root | 195.123.241.49 | tB983y6ZUgT9 | USA | https://profitserver.ru | Kasazhtiklon@yahoo.com | GKcM6PMLI5wi | 5 |
root | 85.204.116.213 | 946𝐴𝑥𝑃4𝑓𝐺𝑥𝐿𝑢𝑒𝑛6𝑅3𝐿 | RO | https://ℎ𝑜𝑠𝑡𝑚𝑎𝑧𝑒.𝑐𝑜𝑚 | Kasazhtiklon@𝑦𝑎ℎ𝑜𝑜.𝑐𝑜𝑚 | GKcM6𝑃𝑀𝐿𝐼5𝑤𝑖 | 5 |
root | 104.161.32.118 | 7xgOhPHb9MNTKAk | USA | https://indovirtue.com/ | Kasazhtiklon@yahoo.com | GKcM6PMLI5wi | 6.6 |
root | 88.150.180.33 | 2𝑂1𝐹𝑣𝑞1𝑔𝑘9 | UK | https://𝑤𝑤𝑤.𝑣𝑒𝑒𝑏𝑙𝑒.𝑜𝑟𝑔 | Kasazhtiklon@𝑦𝑎ℎ𝑜𝑜.𝑐𝑜𝑚 | GKcM6𝑃𝑀𝐿𝐼5𝑤𝑖 | 5 |
root | 45.148.10.143 | 34fhjdgEN3rE5ff | NL | https://dmzhost.co/ | Kasazhtiklon@yahoo.com | GKcM6PMLI5wi | 6 |
The columns seem to show the username (root), the IP address, and the password to access an element of the infrastructure (VPSs). The user Kasazhtiklon@yahoo.com in this case, is the user registered with the VPS provider. While the email addresses that appeared the most (top) are related to the infrastructure, there are 322 addresses that were seen only once in a chat. These are the ones in which attribution is likely to happen. One example comes from the actor zulas who shared his personal jabber account begemot_sun@jabber.ru.
Using this username ('begemot_sun') we easily discovered it is linked to an account on 'habr.com', on 'github.com', Skype, and others (see screenshot of Google). From those social networks or messaging systems we also discovered his personal email ('loguntsov@gmail.com'). Based on his personal email, much more information can be discovered. All these users from different systems and social networks are linked by either the same email ('loguntsov@gmail.com'), or name (Sergey Logunstov), or birthday ('15th of July'). A Twitter user @trickleaks created the full profile of Sergey Loguntsov [6].
We successfully reproduced this case of zulas/Sergey on the remaining 321 addresses that were seen only once in a chat. However, it is not the purpose of this blog to reveal the real identity of Conti actors. We solely aim to show that this is easily possible. Our observations tell us that the attribution is possible since ‘programmers’/’hackers’/’computer scientists’ that decide to join any ransomware gang will keep their public-face life, meaning they use their personal accounts in a ‘work environment’. So, from the moment they share one type of personal information (i.e., email) much more information can be discovered.
4. What IP addresses and URLs (HTTP and HTTPS) are part of the infrastructure of the gang?
This section is divided into three parts. The first section is about the IP addresses that appeared most frequently in the chats, the second and third part elaborate on the top HTTP and HTTPS URLs, respectively.
4.1 IP addresses
We observed 679 distinct IP addresses in the chats. Subsequently, we listed the top 10 IP addresses that appeared most frequently in the chats. We enriched those IP addresses with its autonomous system information (AS name), the country code (CC), and the hostname. Finally, we analysed the context of the chats where those IP addresses appeared to infer their purpose to the Conti gang.IP address | Freq. | AS Name | CC | Hostname | Inferred Function | |
1 | 173.232.146.236 | 62 | EONIX-COMMUNICATIONS | US | server.ekmanqroup.com | VPS |
2 | 82.146.37.128 | 60 | THEFIRST-AS | RU | server49.com | Malware hosting |
3 | 173.232.146.199 | 36 | EONIX-COMMUNICATIONS | US | irate.joystell.com | VPS |
- | 127.0.0.1 | 34 | - | - | localhost | TOR proxy |
4 | 212.129.41.246 | 28 | Online SAS | FR | enemysky.com | - |
5 | 31.214.240.203 | 28 | ACTIVE-SERVERS | DE | - | Loader |
6 | 51.89.125.28 | 23 | OVH | FR | ip28.ip-51-89-125.eu | Contains the bot |
7 | 107.174.192.219 | 19 | COLOCROSSING | US | 107-174-192-219-host.colocrossing.com | Malware hosting |
8 | 18.188.194.80 | 14 | AMAZON-02 | US | ec2-18-188-194-80.us-east-2.compute.amazonaws.com | Bot |
9 | 162.244.81.87 | 14 | SERVERROOM | US | ns4.1xxxhost.net | VPS |
10 | 94.140.115.219 | 14 | NANO-AS | LV | - | Malware hosting1 |
Based on the chats we concluded that Conti uses servers for various tasks, which include VPS-servers, generic command-and-control (C2) servers, VPN servers and malware hosting servers. The first group consists of generic VPS-servers (e.g., 173.232.146.236, 173.232.146.199, 162.244.81.87). Even though most servers that fall into different categories are also hosted on VPS-servers, this group includes servers which are hired by a specific actor for an unstated purpose. These servers are supplied by a few actors and provided to other actors in the group. The buying actor requesting for a VPS must pay for this usage.
The purpose is not included in the messages. We find it likely that actors use this to provide support in any phase within their attacks and for their own development. The second group contains the generic C2 servers (e.g., 51.89.125.28, 18.188.194.80). These servers are purposed to control infected victim machines and offers the possibility to let trojans and backdoors connect, after which they can receive instructions. The third group contains VPN or proxy servers (not included in the table). Messages exist in which actors ask Infrastructure Supplying actors for VPN server credentials.
These servers may be used by actors to hide their origin location during attacks, and aid in their persistence in a victim’s environment. The fourth group consists of malware hosting servers (e.g., 94.140.115.219, 82.146.37.128 and 107.174.192.219). These servers host a website containing malicious downloads. These may be used by dropper malware or phishing e-mails to install backdoors on victim machines controlled by Conti. In the section below, we attribute the IP 107.174.192.219 to host TrickBot malware.
4.2 HTTP URLs
We observed 389 distinct HTTP URLs. Surprisingly, all top 10 point to Webpages without a domain name (but an IP address). This may suggest that the gang does not want to make it publicly available but keep it only accessible to the people that have the IP address.
HTTP URL list | Freq. | Inferred function | |
1 | http://107.174.192.219/images/redcar.png | 19 | Trickbot dropper |
2 | http://107.174.192.219/images/cursor.png | 19 | Trickbot dropper |
3 | http://107.174.192.219/images/imgpaper.png | 19 | Trickbot dropper |
4 | http://104.161.32.109/images/shortwave.png | 12 | Trickbot dropper |
5 | http://104.161.32.109/images/longrip.png | 12 | Trickbot dropper |
6 | http://104.161.32.109/images/green.png | 12 | Trickbot dropper |
7 | http://66.70.218.46/images/redcar.png | 10 | Trickbot dropper |
8 | http://66.70.218.46/images/cursor.png | 10 | Trickbot dropper |
9 | http://66.70.218.46/images/imgpaper.png | 10 | Trickbot dropper |
10 | http://204.155.31.175/images/imgpaper.png | 9 | Trickbot dropper |
These top 10 URLs point to 4 IP addresses: 107.174.192.219 (top 1, 2, and 3), 104.161.32.109 (top 4, 5, and 6), 66.70.218.46 (top 7, 8, 9) and 204.155.31.175 (top 10). All URLs contain malware files that are used as backdoors. We managed to link these IP addresses to TrickBot via URLhaus or Malwarebazaar or in a “order granting plaintiffs’ amended motion”. In the context of the chats, these URLs were sent by actor ttrr to multiple actors. Ttrr said that “the IPs are used for different purposes than droppers as well”, such as internal testing as it seems from other conversations on the IPs. Ttrr is involved in the development of TrickBot, as he distributes the IPs of TrickBot droppers, and he said: “you need to reproduce the crash on the trick module, so that the three of us with the driver and the encoder of the module figure out what exactly is crooked”. We later discovered that “three of us” means him (ttrr), mentos and zulas. These last-mentioned two actors were already identified and exposed by a Twitter account @trickleaks (as described in the previous section).
4.3 HTTPS URLs
We identified 5593 distinct HTTPS URLs. The top 10 were already discussed when we talked about the top 10 email addresses. All these HTTPS URLs are related to the infrastructure used by the Conti gang. All of them can be found in conversations between ganesh and stern. These emails had a high frequency because very frequently ganesh seems to update the users, or the email, or the IP address, or the password of these systems.
HTTPS URL list | Freq. | Inferred function | |
1 | https://host-for.net | 98 | VPS |
2 | https://my.flaunt7.com | 94 | VPS |
3 | https://profitserver.ru | 94 | VPS |
4 | https://cryptoho.st/ | 94 | VPS |
5 | https://deinserverhost.de | 92 | VPS |
6 | https://germanvps.com | 92 | VPS |
7 | https://www.centohost.com | 88 | VPS |
8 | https://hostmaze.com | 88 | VPS |
9 | https://www.vpsroom.com | 84 | VPS |
10 | https://www.veeble.org | 76 | VPS |
Overall, in this section we’ve learned more than IP addresses and URLs used by the Conti gang. We have for example learned about the companies chosen by the Conti gang to host their infrastructure and that their infrastructure is widely distributed. The information in this section can be used to, for example, monitor IP addresses with malicious behaviour coming from the companies used by Conti to host their infrastructure.
5. How many Conti victims are there and who are they?
We have three ways to answer these questions. The first is related to the bitcoin records found in the chat (i.e., victims that paid the ransom). The second way to answer these questions is based on victims who were exposed on the Conti blog that can be accessed on the dark Web. These are victims that did NOT pay the ransom and therefore got their data leaked [7] . Finally, the victims that were explicitly found in chats can be looked at to answer these main questions. The sections below cover these three ways and summarises the correlation between them.
5.1. BITCOIN WALLETS
We observed 261 distinct Bitcoin wallets. The top 10 wallets that contained the most transactions are listed below. We also present the number of times it was observed in chats (freq.), the number of Bitcoin transactions using this wallet, the amount in Bitcoin (BTC) received/sent to another wallet(s), and the estimated value. Bitcoin Explorer (at https://blockchain.info) was used to discover how much was exchanged by each Bitcoin wallet. As the values of Bitcoin fluctuate over time, we used the average values from January to December of 2021, €40.228,40 (from https://www.investing.com/crypto/bitcoin/historical-data).
Wallet | Freq. | Transac. | Exchanged [BTC] | Exchanged [€] | |
1 | 1EZY2exVq9LvbwBaXWPoR7bYhfNRBXC3m5 | 10 | 21 | 575,30616613 | € 23.143.646,57 |
2 | 3A99TqWrJ1cVDWKnTyqjAGXMcUFa3Rt2TZ | 2 | 33 | 215,26621418 | € 8.659.815,37 |
3 | 1G9Zwp2cBdrM8o3wpactcqrw6NC3xGEm1o | 1 | 17 | 125,00000000 | € 5.028.550,00 |
4 | 1AXiwETqqQoA52Jk5CmJkbAPuW8nR7VUYz | 1 | 39 | 111,04859631 | € 4.467.307,35 |
5 | 3AzWagPyw6fK8zhXhnyiHo57AN4Xqnt7rw | 1 | 2 | 105,00000000 | € 4.223.982,00 |
6 | bc1qfht3lj9hda30pv4mqqkklxjylmm9jma0k4ssag | 1 | 2 | 81,40000000 | € 3.274.591,76 |
7 | bc1qy0gz9dhhck0nwg2nm5feeufczjms7m0vyvsmss | 1 | 14 | 76,24337500 | € 3.067.148,99 |
8 | 19UNe82ipWkoqfBx35Km1HpJPp29s6qKno | 1 | 5 | 41,96667162 | € 1.688.252,05 |
9 | bc1qa225n5kp0e73n4hthea3fqv4rgvwuzx65wldxv | 2 | 2 | 37,50000000 | € 1.508.565,00 |
10 | 1NkKgMkX3vud7uo999d8GuExkkwpNwZDYa | 1 | 4 | 35,60000000 | € 1.432.131,04 |
Based on our enriched data, we observed all ranges of values exchanged in Bitcoin wallets. A wallet usually has 2 or more transactions, one is from the victim to the Conti gang and the second is from the gang to one or more wallet(s) also from the gang. It is very difficult to make attribution from the bitcoin wallets. However, our experience says that victims are charged anywhere from hundreds of thousands to ransoms of even millions of Euros. There are 33 wallets that exchanged more than €100k.
We managed to identify several victims by comparing the chat timeline to the wallets, for example the wallet on the 10th position of the top 10. This wallet (10th position) was found in a chat between professor and stern (on 23/09/2020). On the same day, this wallet received 27,5 BTC (more than 1M Euros). The conversation explicitly exposes the name of the company that fell victim to the attack. On the news, in the same period, this victim confirmed a data breach (but not a ransomware, and it was also not mentioned that they paid the ransom). These findings prove that the correlation between the chats and bitcoin wallets can be used to review which victims paid the ransom.
5.2. VICTIMS IN THE CHATS
Within the chats, we identified (only) 33 victims. Possibly we miss some of the victims in the data. The victims were found mainly in conversations involving the actors tramp or stern. We were able to validate some of these victims on news items found via Google or on Conti news website (described in the next section). Surprisingly, Conti actors exchanged valuable information on victims, such as: the number of servers, the number of employees, the year revenue, even the antivirus (AV) used, among other information. We decided to omit the conversation to avoid exposing companies that fell victim to the Conti group.
5.3. VICTIMS ON THE CONTI NEWS WEBSITE
Any ransomware gang has a (publicly available) Webpage on their victims. The Conti gang maintains their Webpage on the dark Web [7] accessible via the TOR browser. Note in the screenshot below that for each victim they display some information about the victim and the percentage of the data published/leaked. Anyone with this URL can see the victims and their data. On the time of the analysis, 11/03/2022, we observed 309 victims on the Conti news Website going back to 29/7/2020 (one month after the first chat record leaked). Northwave's experience shows that only victims that did not pay the ransom got their data leaked (at some point in time). Therefore, there is no overlap between victims on their website and victims that paid BTC.
As expected, there is an overlap between victims on their website and victims in chats. We managed to validate 14 victims in both sets (remaining 19 victims identified only on the chat). This is remarkable, because six of them have a URL pointing to the blog, but the page is not there (anymore). Our hypothesis is that these six victims eventually paid the ransom and were removed from the Conti news Webpage.
Merging all findings from the bitcoin wallets, chats, and the Conti news webpage, we observed around half a thousand of victims between mid-2020 till early-2022. We are surprised to observe that the data leak didn’t slow down the gang. Since the data was leaked there are updates on the Conti news website that their operation didn’t stop and more victims are still suffering from the attacks.
6. Closing message
In this blog, we analysed leaked data related to the Conti ransomware gang. Our goal was to show what meaningful information we can deduct from the data. We focused our efforts on answering questions related to Conti’s members (their roles and their real identity), the infrastructure used by them, and their victims. Instead of displaying all findings, we display the most relevant information per topic. We also focus on explain how we arrive at those conclusions. The reason for that is approach is two-fold: firstly, to protect the identity of victims and secondly, to facilitate further analysis based on the data.
Acknowledgements
We thank the Northwave CERT team for spending hours translating, analysing, enriching, and discussing the data, in special: Jair Santanna, Stephan Benjamins, Vlada Kulish, Bastiaan Weijers, Ralph Horn, Patrick van Looy, Erwin Maas, and Pim Takkenberg.-
Sources
- https://northwave-security.com/when-the-hackers-get-hacked-part-1-a-blog-series-unveiling-the-conti-ransomware-family/
- https://github.com/NorthwaveSecurity/complete_translation_leaked_chats_conti_ransomware
- https://share.vx-underground.org/Conti/
- https://krebsonsecurity.com/2022/03/conti-ransomware-group-diaries-part-i-evasion/
- https://noticeofpleadings.com/trickbot/files/DefaultJudgment/2021.05.21%20Trickbot%20ECF%2062%20NoH%20re%20Motion%20for%20Default%20Judgment%20&%20PI%20Order.pdf
- https://twitter.com/trickleaks/status/1499740068281475073
- http://continewsnv5otx5kaoje7krkto2qbu3gtqef22mnr7eaxw3y6ncz3ad.onion/